For decades, transportation has relied heavily on human decision-making and infrastructure limitations. Traffic management systems haven’t kept pace with urban growth, leading to congestion and safety concerns. Additionally, traditional vehicle design prioritises human control, often neglecting the potential for technological assistance.
The evolution of AI in mobility
Artificial Intelligence (AI) is poised to revolutionise the mobility landscape, shattering long-held assumptions about the limitations of computer systems in tackling complex problems. This transformation hinges on the capabilities of machine learning (ML) and artificial intelligence, enabling machines to perceive (see, listen), interpret, predict, and comprehend – mimicking human capabilities. The notion that machines lack these essential faculties is being debunked by the advancements in AI technology.
Let’s take a closer look at some promising areas where AI is being leveraged to transform the way we move.
Streamlining Demand-Responsive Transport in sparsely populated areas
Demand-Responsive Transport (DRT) offers an innovative approach to traditional public transportation, particularly benefiting sparsely populated areas with lower demand, offering a more flexible and cost-effective alternative to fixed-route buses.
Transforming Public Transport NEMI’s AI-Powered DRT Innovations
NEMI, a market leader in DRT solutions in Spain, leverages AI within a multivariable parametrised optimization tool. This AI-driven approach allows NEMI to simulate demand-responsive mobility services using various optimisation algorithms tailored for shared mobility. This results in broader route coverage, improved accessibility for a wider audience, more accurate passenger information, reduced travel times, and streamlined digitalisation of public transport services.
AI algorithms are being developed in the context of JULIA, a Horizon Europe project coordinated by Factual that aims to enhance public transport’s reliability, safety, and sustainability using EU Space Data and Services.
These algorithms, fed by Origin-Destination data from Galileo-enabled devices, will be exploited to provide highly personalised bus travel solutions using Machine Learning. This approach will support mobility planning, select the most appropriate mobility offers, and provide more accurate real-time pick-up and arrival information while optimizing the overall operation of Demand Responsive Transit systems.
AI in mobility will also be used to improve the vehicle map-matching process, where topology-based techniques will be combined with Machine Learning capabilities using similarity indicators to significantly reduce geotagging errors, using it for the more precise location of bus dynamic stops in DRT.
NEMI leverages Galileo services, the European Global Navigation Satellite System. Galileo’s precise and reliable positioning information is crucial for DRT. It enables accurate planning and real-time adjustments of routes, minimising friction between users requesting rides and the available buses that can serve their needs. This integration further enhances the efficiency and responsiveness of shared mobility services, ultimately benefiting a larger portion of the population.
Automatic recognition of cycling infrastructure attributes in safety assessments
Lane Patrol is a first-to-market software tool that maps and assesses the safety of cycling infrastructures using the CycleRAP methodology. By focusing on evidence-based risk assessments, primarily extracted from cycling infrastructure images (or video footage), the CycleRAP model helps identify high-risk areas without relying exclusively on past collision data.
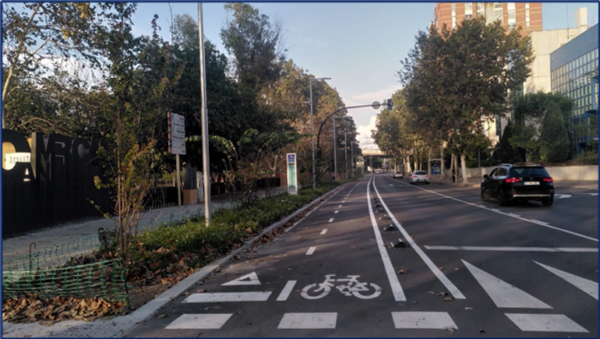
Cycle Lane in Barcelona
Up until now, the process of collecting and analysing the images for CycleRAP has been mainly manual, which involves significant hands-on work and can be time-consuming, plus prone to errors. Building on computer vision, Lane Patrol is exploring techniques to automate parts of this process, simplifying and accelerating the CycleRAP evaluation process. Deep learning models, such as deep neural networks, are a type of Artificial Intelligence (AI) capable of processing and interpreting complex data with high accuracy. At the image level, they are capable of performing complex tasks such as image classification (to determine lane types, the presence of tram rails, or whether there is light segregation, that is, if the image contains curbs, bollards, etc.); object detection (capable of detecting many types of road defects, persons, such as pedestrians, other light vehicles, etc.); object tracking (of other cyclists, pedestrians, cars, etc.); or semantic segmentation (for lane detection, including the presence of proper delineation, etc.).
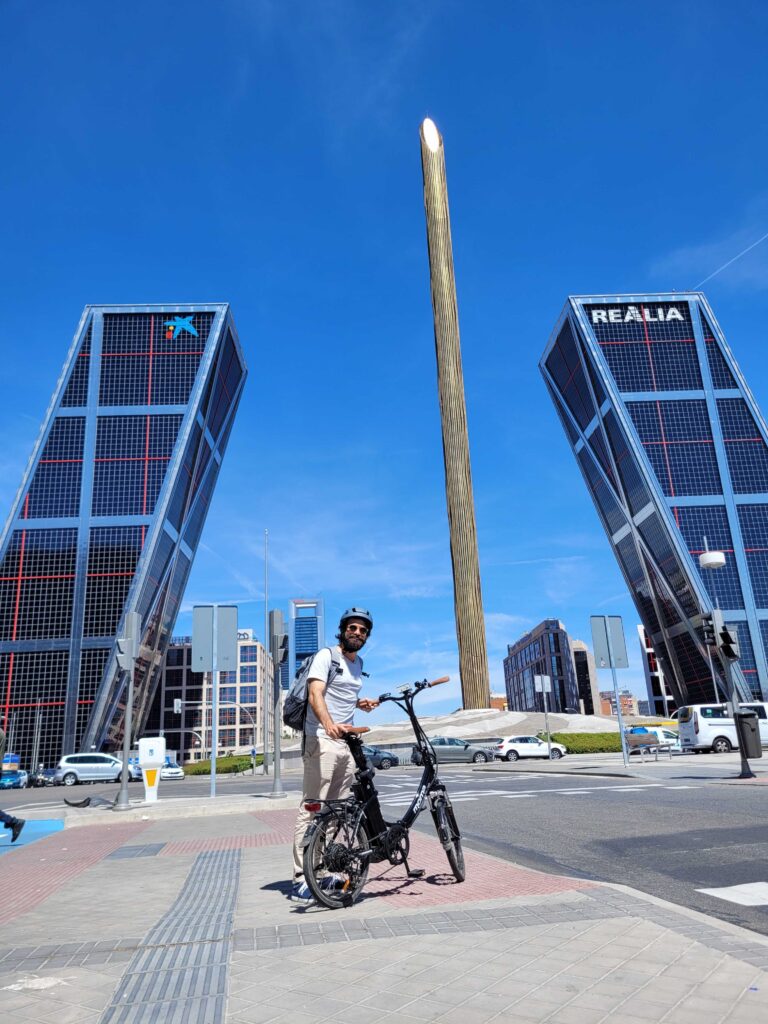
Lane Patrol Cycling Infrastructure Assessment in Madrid
All these methods are suitable for classifying the attributes of the CycleRAP method. Lane Patrol uses an AWS AI algorithm to blur faces and license plates before uploading the images to the server for further processing, therefore ensuring privacy protection. It is worth noting that the integration of computer vision, particularly with learning algorithms, enables the CycleRAP attribute classification to improve over time: as more data is gathered and labelled, the systems will become increasingly accurate.
CycleRAP requires acquiring images of a cycle lane down to every 10 metres. The accurate calculation of this 10-metre distance is solely based on the positions calculated by the GNSS receiver integrated into the acquisition device (Lane Patrol smartphone app), and hence, accuracy and positioning availability are key.
Errors in positioning calculations can lead to distance calculation errors and, consequently, image results that do not meet the criteria for a successful assessment. In this scenario, the use of multi-constellation GNSS receivers with Galileo has proven to be a good approach, especially in urban environments where the number of satellites in view is constrained by surrounding buildings. This use case is also part of the JULIA project.
AI in mobility: connected cars, a double-edged sword
Connected cars are revolutionising the way we navigate the roads. From lane departure warnings to automatic emergency braking, AI-powered features in cars (ADAS) are making driving safer by helping us avoid common mistakes. These technologies act as a virtual co-pilot, constantly monitoring the road and the driver’s actions. By analysing sensor data and recognising potential hazards, AI can intervene and take corrective actions, mitigating the risks associated with human error.
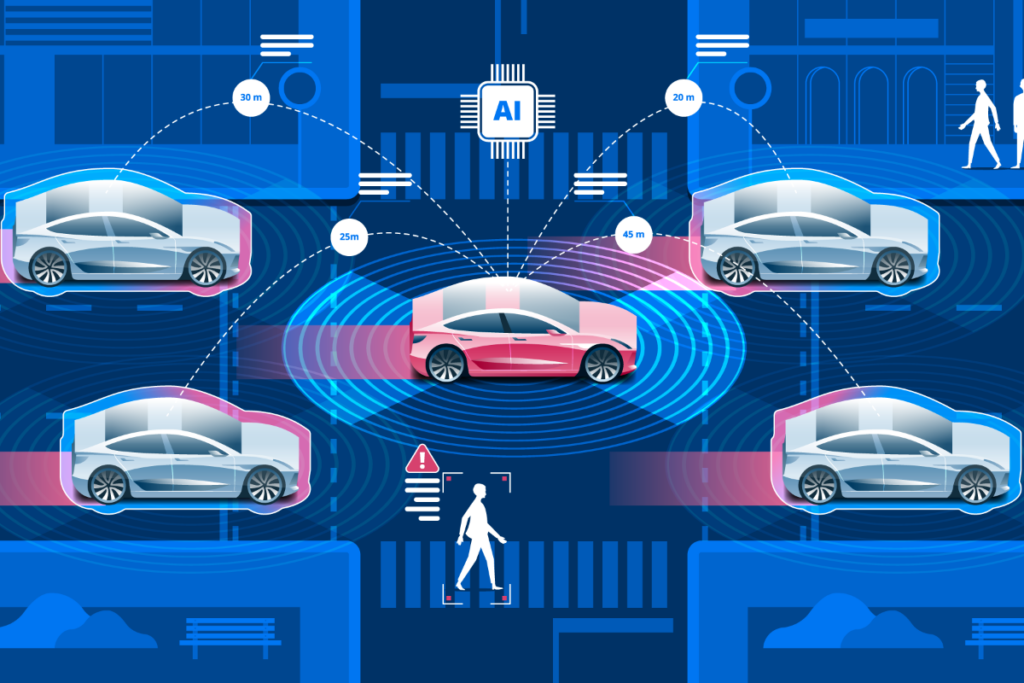
AI in mobility: AI-powered features in cars (ADAS) are making driving safer
The evolution of automation levels
Level 1-2 automation, through ADAS features, is already offering significant safety benefits and becoming mainstream in modern cars. The conversation, however, is expanding to encompass Level 3 cars, with some models already available in the market. Level 4 remains a utopia, with its potential benefits that must be carefully weighed against the significant complexity and cost of implementation. Some even argue that Level 4 technology might be a “solution looking for a problem”. Moreover, while Level 3-4 cars, aka Autonomous Vehicles (AV), raise the exciting prospect of a world with significantly reduced traffic accidents and a more accessible transportation system, it also necessitates a closer look at the potential risks and challenges associated with handing over such a critical task to AI.
Public Trust and Legal Challenges
A significant hurdle lies in public trust. Many people are understandably apprehensive about relinquishing control of their vehicles to AI. Addressing safety concerns and fostering public trust through rigorous testing and transparent regulations will be crucial. Additionally, the legal framework surrounding AVs is still under development. Clear and comprehensive regulations are needed to ensure safety and define liability in case of accidents.
Cybersecurity and Privacy Concerns
Finally, as cars become increasingly connected, the risk of cyberattacks rises. Robust cybersecurity measures are essential to protect CAVs from hacking and ensure safe and reliable operation. In our recently published white paper on cybersecurity and smart mobility, we dive into how AI can be used to address cybersecurity issues in smart mobility and connected cars. For example, AI algorithms can analyse vast amounts of data from connected cars (sensor data, network traffic) to identify unusual patterns that might indicate a cyberattack, like unauthorised access attempts or suspicious changes in vehicle behaviour, or to analyse vehicle data to predict potential security vulnerabilities before they are exploited, allowing for proactive maintenance, and patching of software flaws. On the other hand, training AI algorithms in CAVs necessitates the collection of a vast amount of user data, including driving habits, location information, and potentially even biometric data. This raises serious privacy, as well as ethics considerations (such as fairness in decision-making by AI algorithms in CAVs).